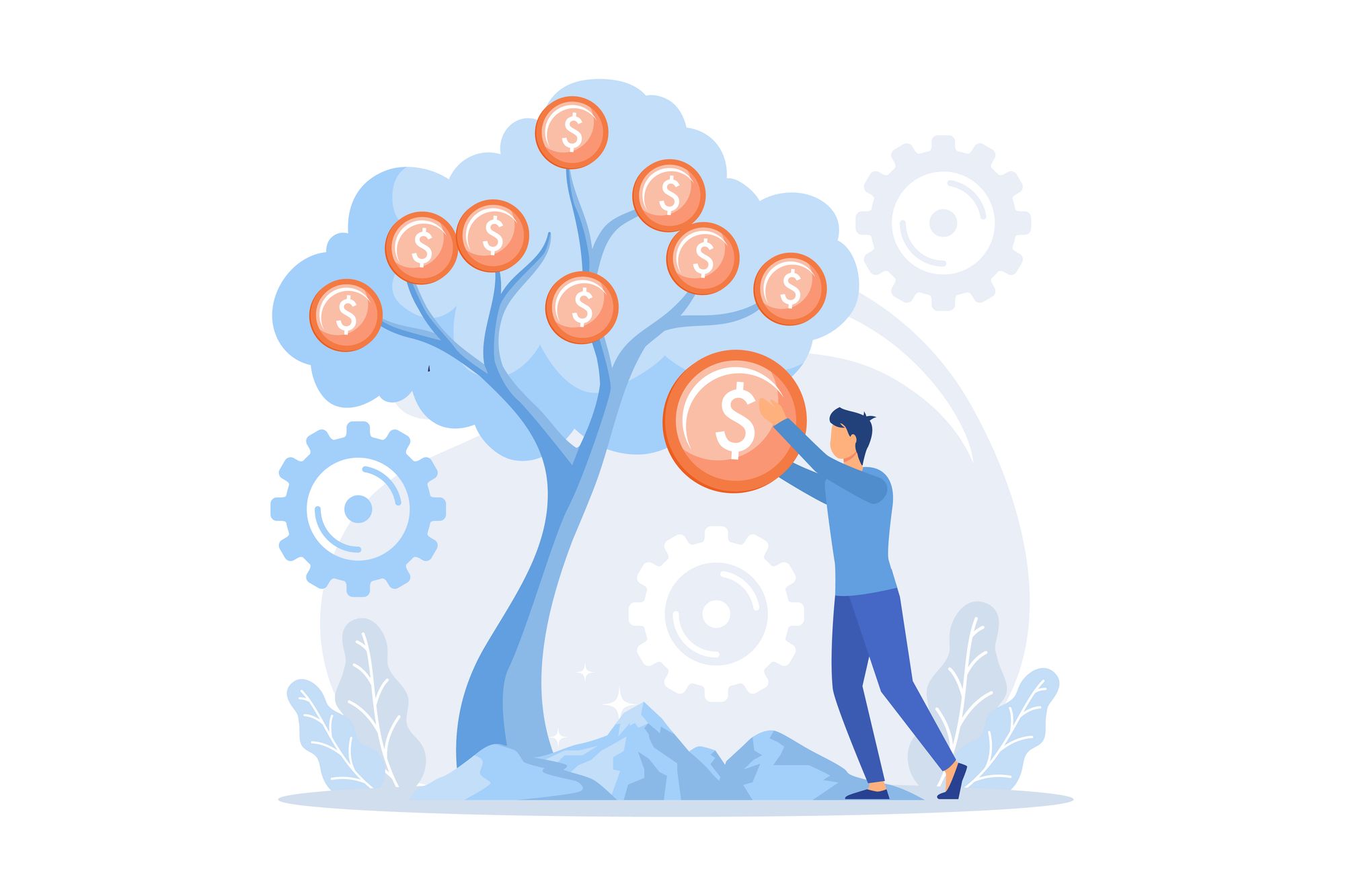
π Definition
A basket decision tree, also known as BDT, is a shopper basket analytical tool used by Retailers to discover relationships and patterns among their Loyal & Non-Loyal Shoppers.
This analytical technique sheds light on sales variations driven by the buying behavior of customers, helping Retailers understand the reasons for sales variations, whether they are influenced by spending or foot traffic.
π§ͺ Case studies and example of Basket Decision Tree
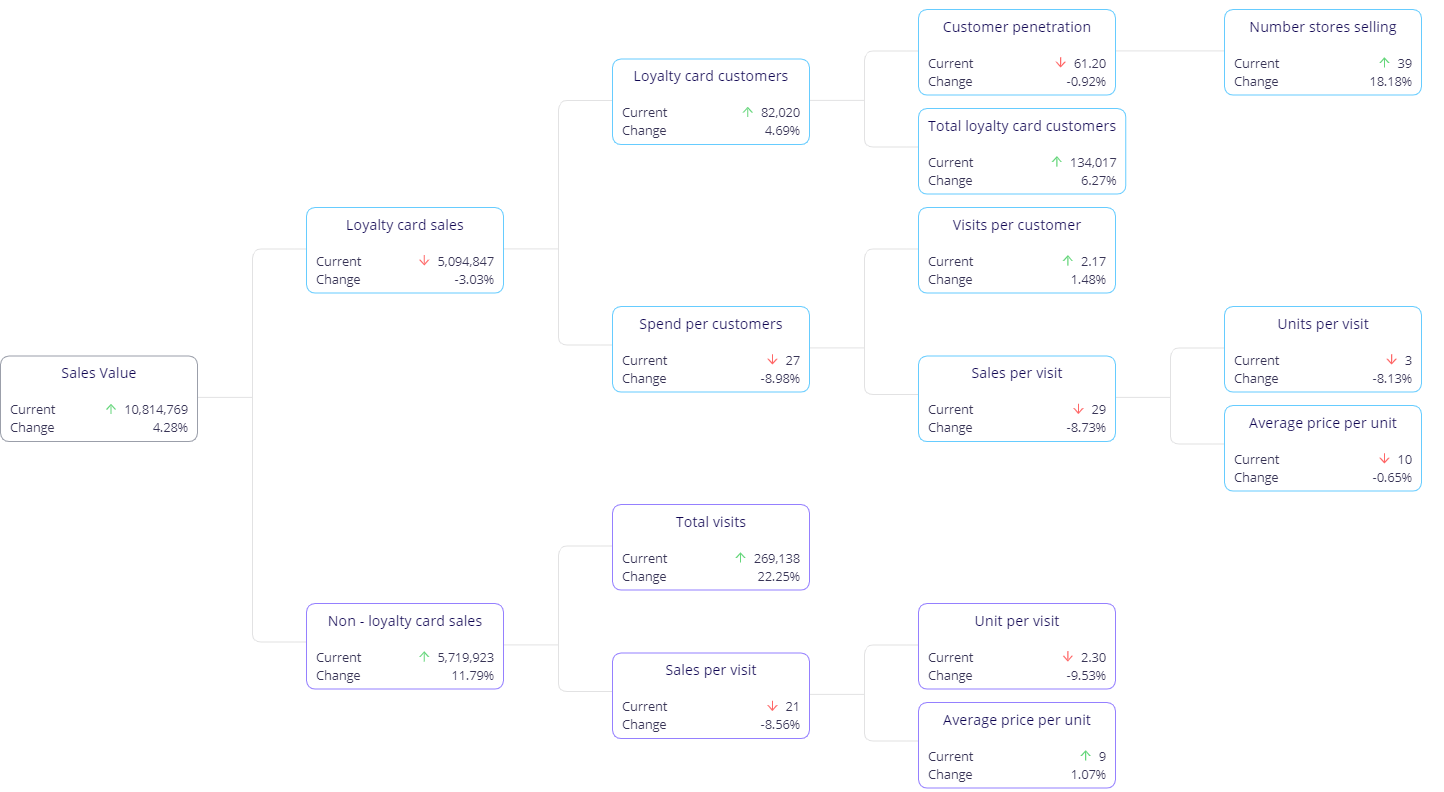
For example, let's consider the above basket decision tree analysis. We can observe the following trends:
- Sales value is growing at a rate of +4.28%.
- Loyalty card sales have decreased by -3.03%, while Non-loyalty card sales have increased by +11.79%.
- The increase in Non-Loyalty carded Shoppers' sales is primarily due to a rise in total visits or foot traffic, which has increased by +22.25%. This increase in traffic compensates for a decrease in Basket Value by -8.56%.
- Conversely, Loyalty card Shoppers have experienced slow growth in foot traffic, with an increase of only +4.69%. This, combined with a decrease in basket value by -8.98%, has led to a decline in sales for this shopper group.
- The main root cause of the decline in both shopper groups can be attributed to a decrease in units per basket, indicating that shoppers are purchasing lesser quantities.
In conclusion, we observe a decrease in quantities purchased by both shopper groups. However, the higher foot traffic generated by Non-Loyalty carded Shoppers' supports total sales growth.
Based on these insights, Retailers can optimize their commercial offerings and tailor their marketing strategies accordingly. They can choose to focus on generating foot traffic from Loyalty card Shoppers or aim for a global increase in quantities purchased by all shoppers.
Please note that this is a generalized example, and the specific insights and recommendations would depend on the actual data and context of the analysis.
βWhat is used for
Once the Basket decision tree is constructed, Retailers can utilize it for various purposes.
- Retailers can gain insight into the factors driving sales variations, such as spending or traffic between their Loyal & Non-Loyal Shoppers.
- The analysis enables Retailers to focus on specific areas to address sales decline or capitalize on sales growth opportunities.
- Understanding the global trends of the shoppers enables retailers to address the key factors and segment their loyal shoppers based on frequency, basket tier, and ABC segments to discover which segments of shoppers to address.
- By analyzing the behaviors and preferences of loyal Shoppers. Retailers can identify opportunities to strengthen relationships and improve customer satisfaction. They can develop Loyalty Programs, personalized offers, and other initiatives to incentivize repeat purchases and foster long-term loyalty.
- Sharing the insights gained from BDT analysis, Manufacturers can work closely with Retailers to align on assortments, promotions, and pricing strategies. As BDT analysis also provides information regarding Penetration, Avg Price per Unit and flexibility of period selection.
How is a Basket Decision Tree constructed and what data inputs are required?
A Basket Decision Tree is typically constructed using advanced analytical techniques that involve processing and analyzing large sets of transactional data. The data inputs required for constructing a Basket Decision Tree usually include information on customer purchases, such as product types, quantities, purchase frequency, and customer segmentation (loyal vs. non-loyal shoppers). Additionally, data on foot traffic, basket value, and sales variations are essential to identify relationships and patterns among different shopper groups. Sophisticated algorithms and statistical methods are employed to segment the data and visualize the relationships, which ultimately results in the creation of the Basket Decision Tree.
What are the potential challenges or limitations of using a Basket Decision Tree in retail analytics?
While Basket Decision Trees offer valuable insights into shopper behavior and sales variations, they come with certain challenges and limitations. One potential challenge is the complexity of interpreting the results, as the relationships and patterns identified may not always be straightforward or easily actionable. Another limitation is the dependency on accurate and comprehensive data; inaccurate or incomplete data can lead to misleading conclusions and ineffective strategies. Additionally, the dynamic nature of consumer behavior and market trends requires Retailers to frequently update their Basket Decision Tree analysis to ensure relevance and effectiveness.
How frequently should Retailers update or revisit their Basket Decision Tree analysis to ensure it remains relevant and actionable?
To maintain the relevance and effectiveness of a Basket Decision Tree analysis, Retailers should consider revisiting and updating their analysis on a regular basis, ideally quarterly or semi-annually. This frequency allows Retailers to adapt to changing market conditions, consumer preferences, and shopping behaviors, ensuring that their strategies and initiatives remain aligned with current trends and opportunities. Additionally, ongoing analysis enables Retailers to monitor the impact of implemented changes and adjustments, facilitating continuous improvement and optimization of their commercial offerings and marketing strategies.
π₯οΈ Make it happen in Ulys Customer Intelligence
How to access Basket Decision Tree in Ulys Customer Intelligence
Step 1: Select the Basket Decision Tree under the Behaviors Menu.
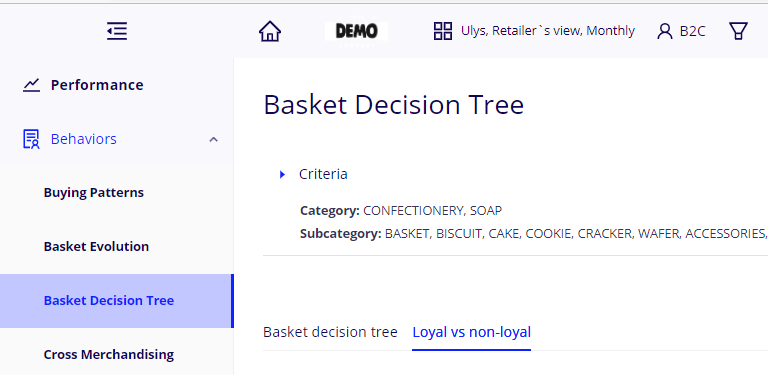
Step 2: Select the Option in the Filter Menu and select the period you want to measure.
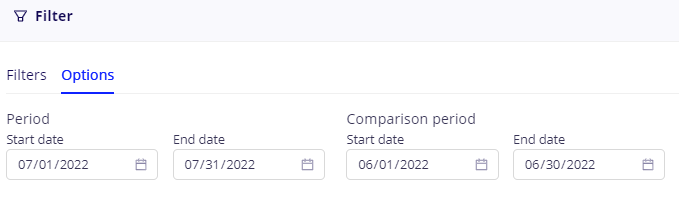
And here it is. The information can be viewed from two perspectives. First is the global summary and the analysis of Loyal vs Non-Loyal Shopper.
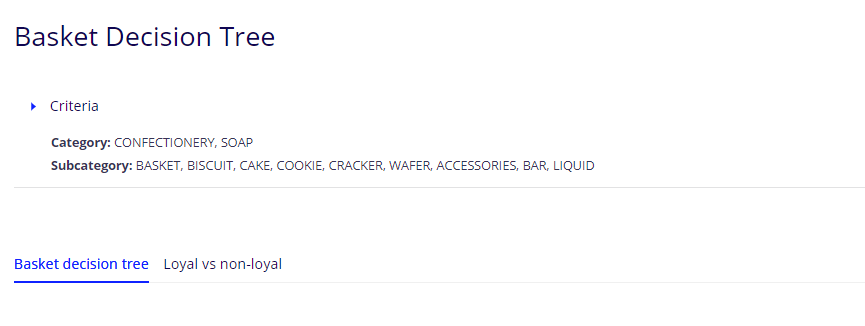
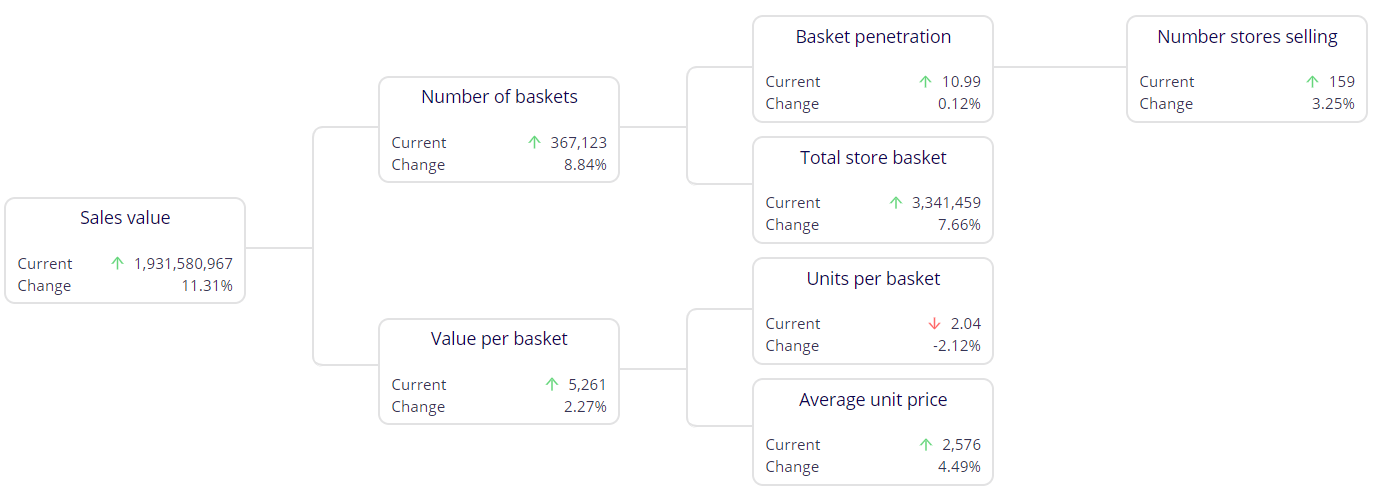
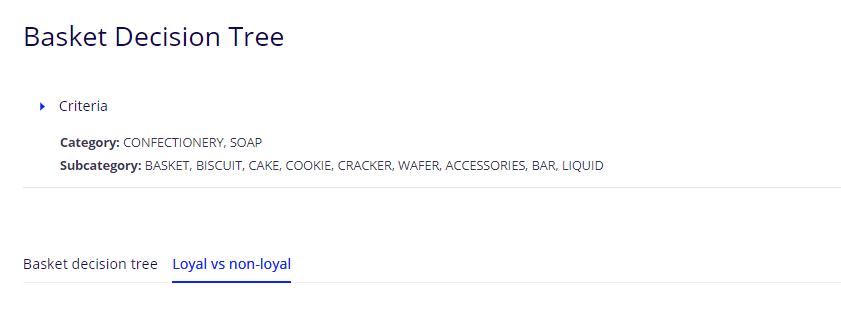
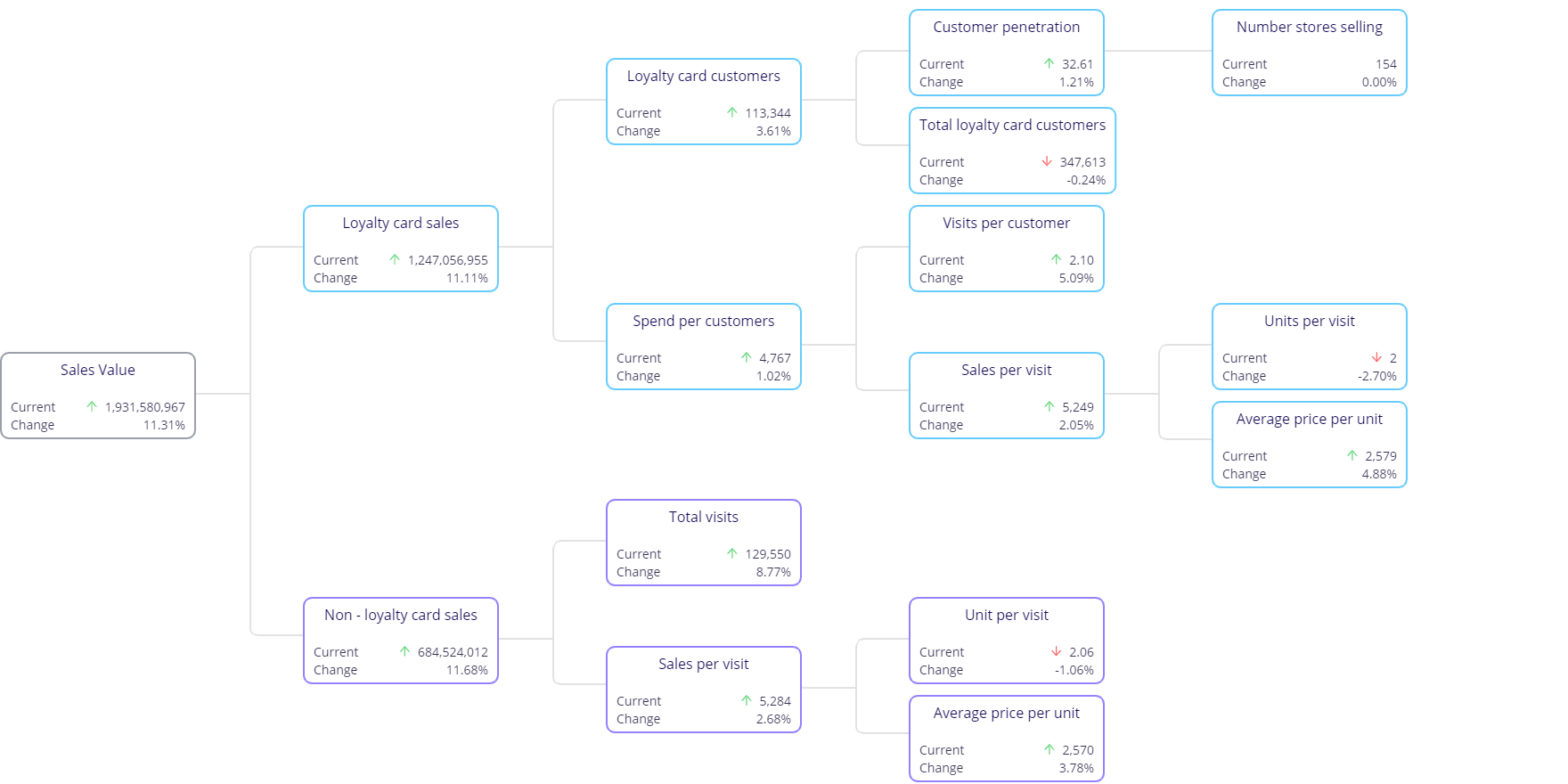